Humanitarian and development
SupR-NTL: Super-Resolution Nighttime Light for Sustainable Development
Leveraging AI and Earth Observation data to improve Nighttime Light (NTL) imagery for assessing energy access
Overview
The SupR-NTL project uses AI-driven techniques to enhance existing NTL imagery at a global level. By improving spatial resolution from 500m to 130m, SupR-NTL offers unprecedented insights into electricity access, energy consumption, and urban growth.
Funded by the European Space Agency (ESA), the SupR-NTL project leverages state-of-the-art Super-Resolution Generative Adversarial Networks (SRGANs) to enhance VIIRS-DNB NTL imagery to 130m in order to better understand electricity access and energy consumption patterns in Sub-Saharan Africa and Southeast Asia. This innovation directly supports progress towards Sustainable Development Goals (SDGs), particularly SDG 7: Affordable, Reliable and Sustainable Energy.
Mastering the challenges of datasets
The SupR-NTL project represents a groundbreaking leap in remote sensing by combining cutting-edge AI technology with underutilized satellite data to address real-world challenges. At its core the developed of a SRGAN, is capable of enhancing NTL imagery from 500m resolution to an unprecedented 130m detail. By integrating data from VIIRS-DNB NTL, Luojia 1-01, and Sentinel-2 satellites, SupR-NTL overcomes long-standing limitations of pixel misalignment, cloud coverage and resolution constraints.
This advanced approach unlocks a finer-grained view of human activity and energy use, revealing the precise identification of underserved communities and small, unlit settlements often hidden in lower-resolution datasets.
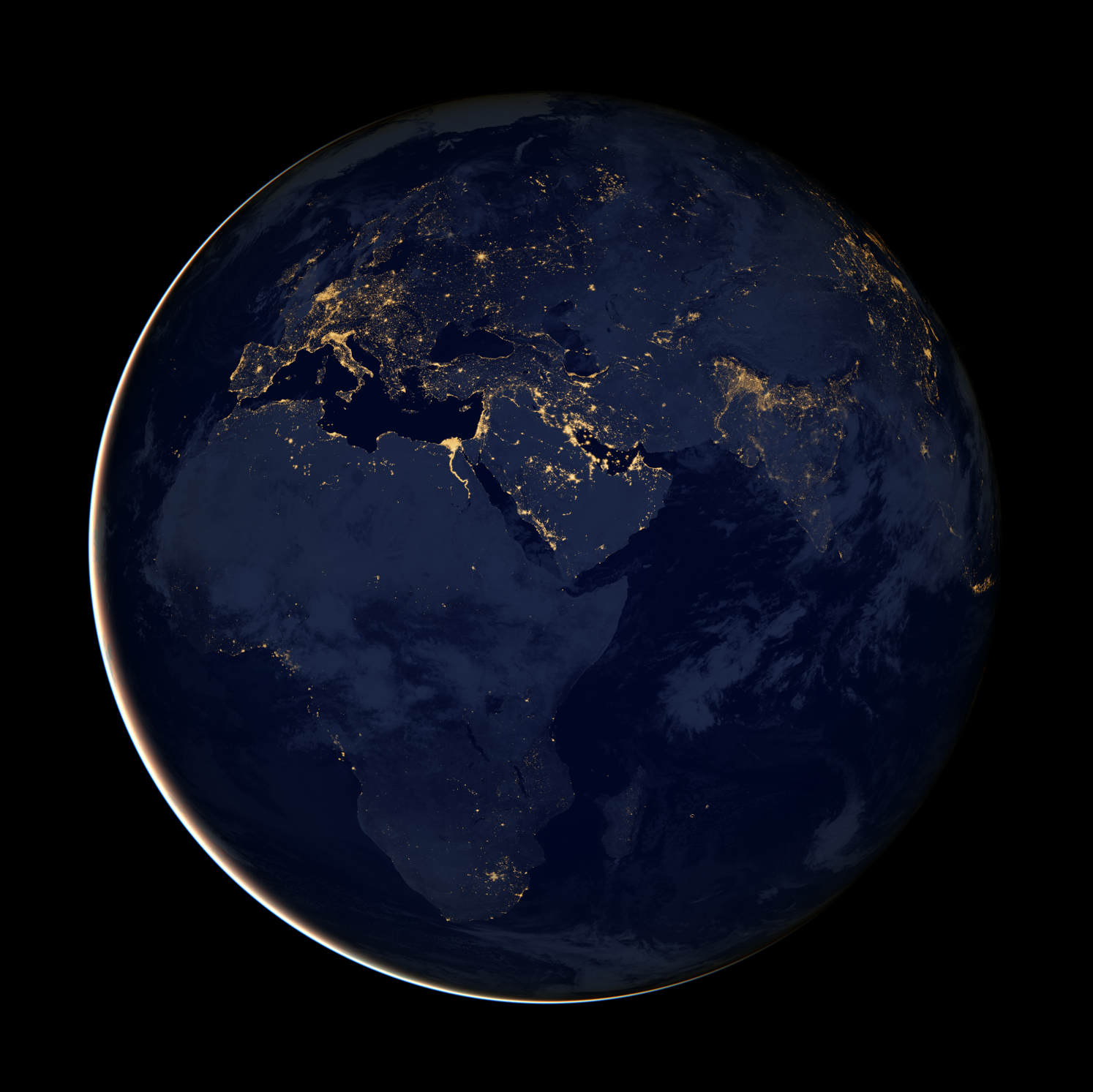
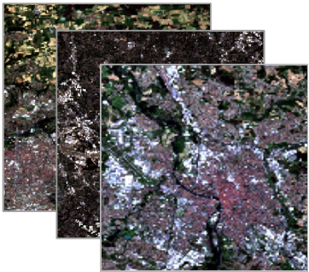
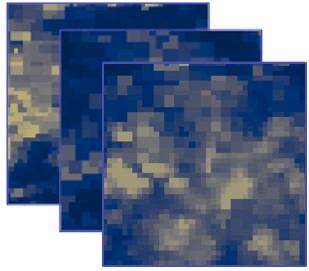
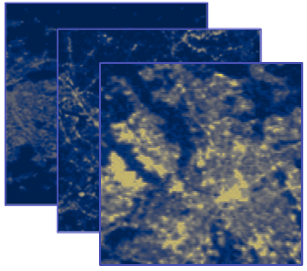
Pioneering AI-Driven NTL Enhancement
The enhanced sharpness of SR-NTL imagery can transform the study of urban and environmental dynamics, providing a foundation for data-driven decision-making. With its improved spatial resolution, SupR-NTL enables more accurate analyses of population distribution, energy consumption, economic activity, and urban sprawl. This refined level of detail empowers policymakers to design tailored interventions and monitor progress with unprecedented precision.
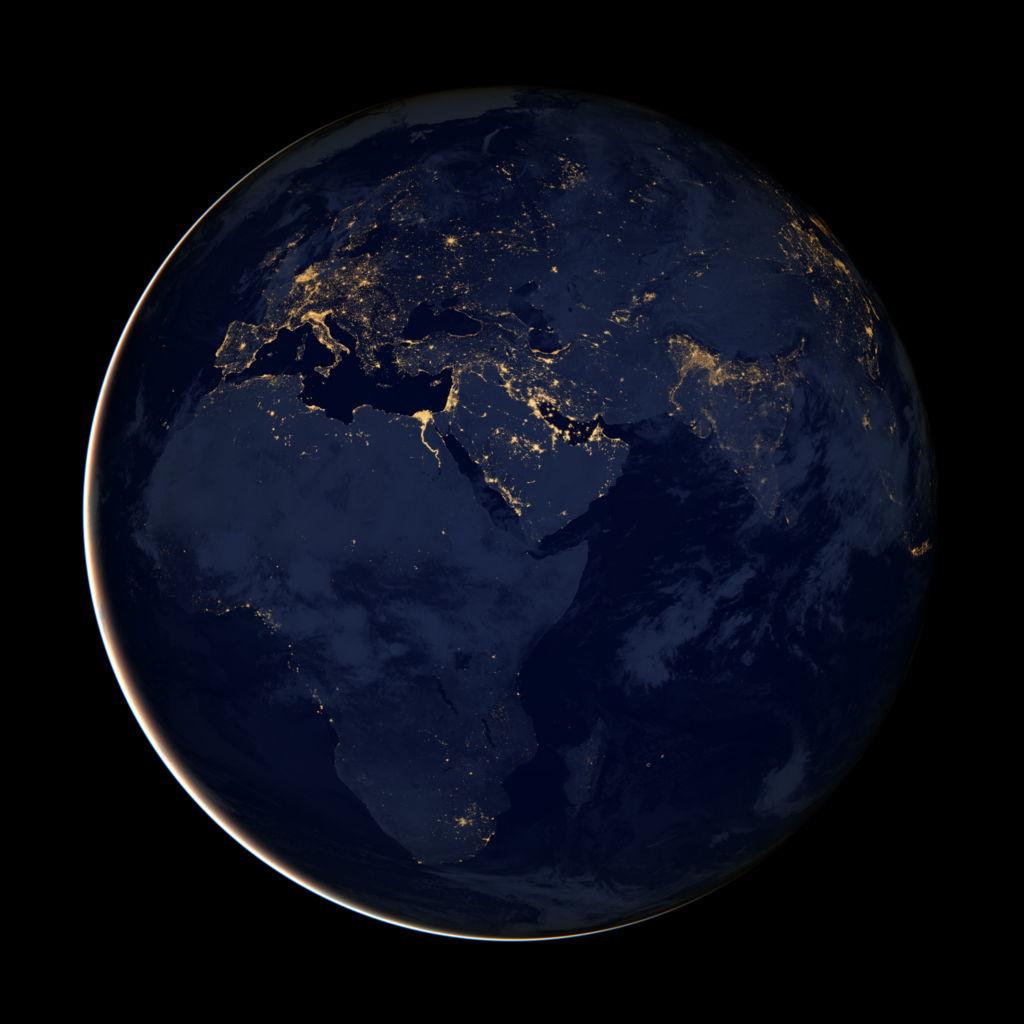
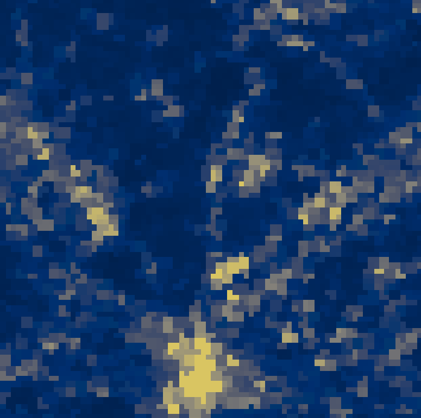
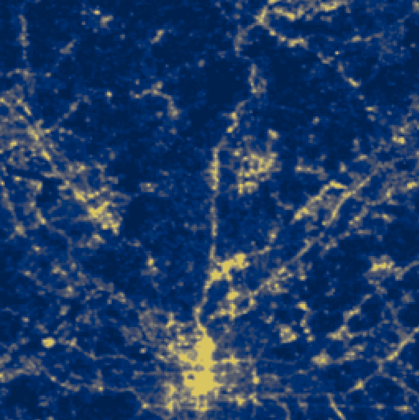
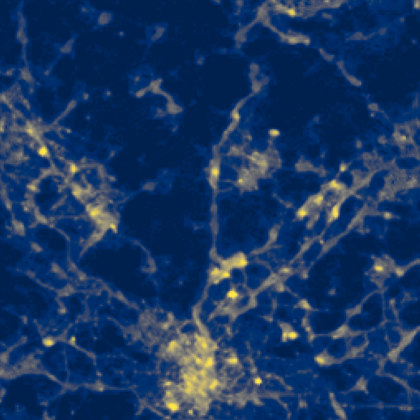
Scaling up our solution
Our SRGAN system is designed to work seamlessly with any VIIRS-DNB NTL data, whether from daily observations or temporally aggregated products such as weekly, monthly, or yearly medians. This flexibility enables allow us to create high-resolution 130 m version of the entire VIIRS-DNB NTL archive from 2016 onwards by exploiting Sentinel-2 imagery.
To ensure our SRGAN system is scalable and reliable, it was tested in +40 major cities worldwide using the 2023 yearly median data for VIIRS-DNB NTL, Sentinel-2 RGB, and Sentinel-2 Red-Infrared (RI).
This achievement has the potential to significantly increase the utility of NTL data by unlocking new application opportunities in urban planning, socioeconomic activities, electricity access assessment, and environmental studies.
Closing the Gap on Electricity Access in Sub-Saharan Africa and Southeast Asia
Sub-Saharan Africa and Southeast Asia remain at the forefront of the global energy access challenge, where millions of people still live without reliable electricity. Despite rapid economic growth in Southeast Asia and targeted electrification initiatives in Africa, disparities persist, particularly in rural and peri-urban areas. The SupR-NTL project provides a powerful tool to address this gap by offering high-resolution NTL imagery that reveals an enhanced extent of energy access at a granular level.
Source: IEA
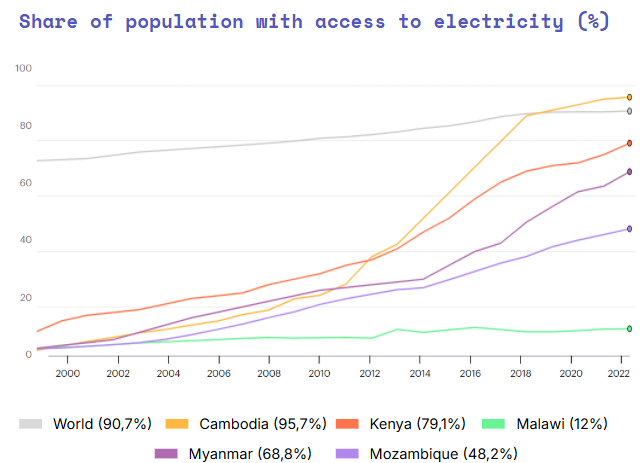
Assessing electricity access
Add context africa + wsf+ examples + change pictures
Explore more use cases
- SupR-NTL: Super-Resolution Nighttime Light for Sustainable Development
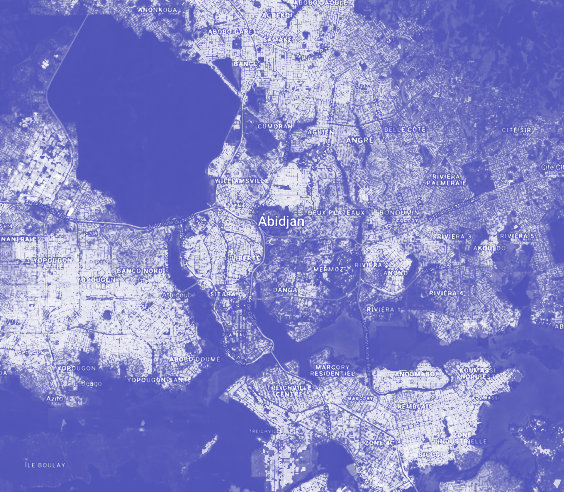
- Community-based cartography & EO for Urban Resilience
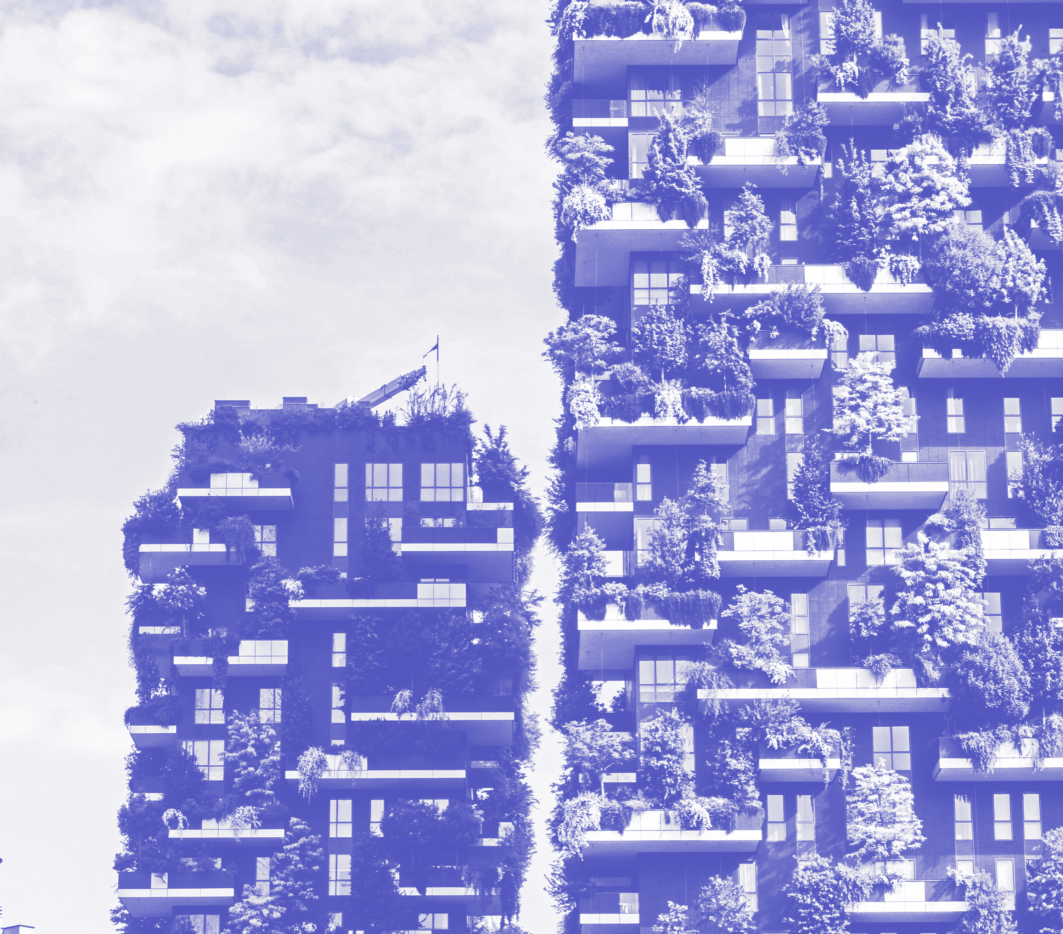
- BEE-AI: Building Energy Efficiency with Artificial Intelligence
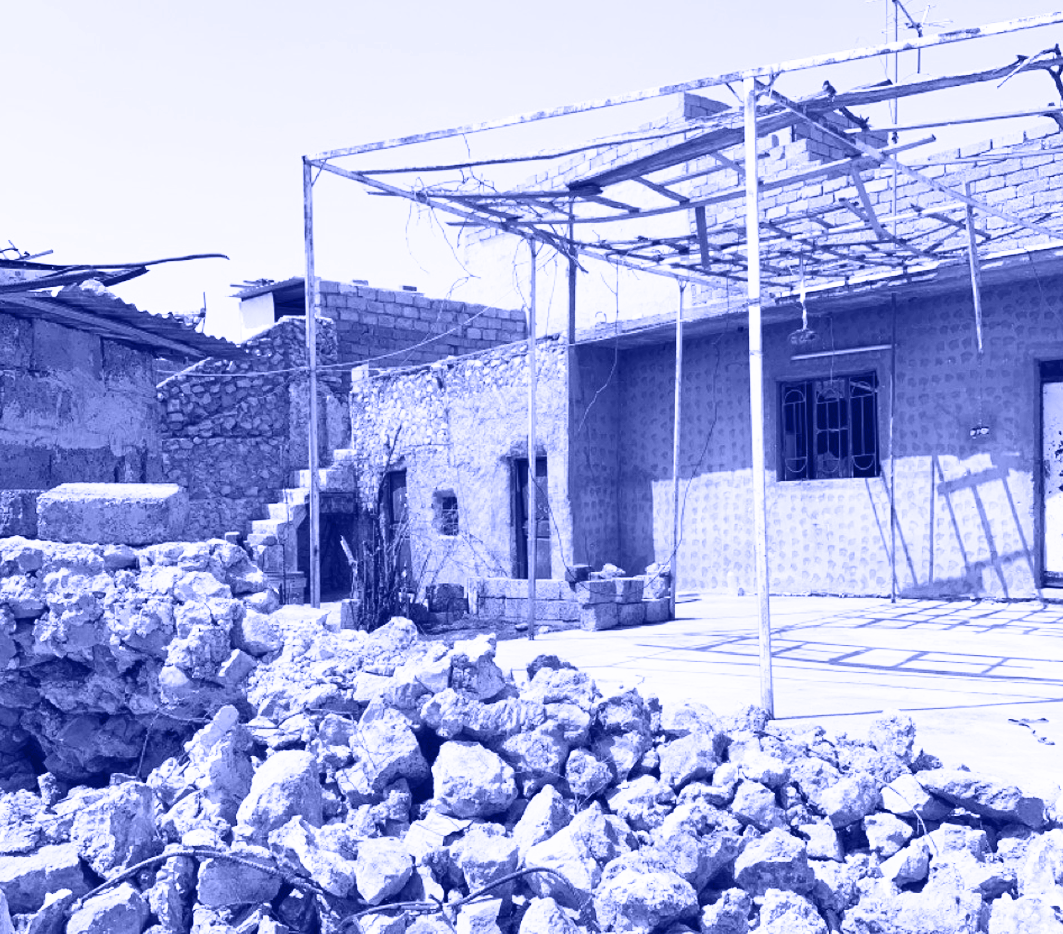
- Mapping the impact of armed conflict through Earth Observation
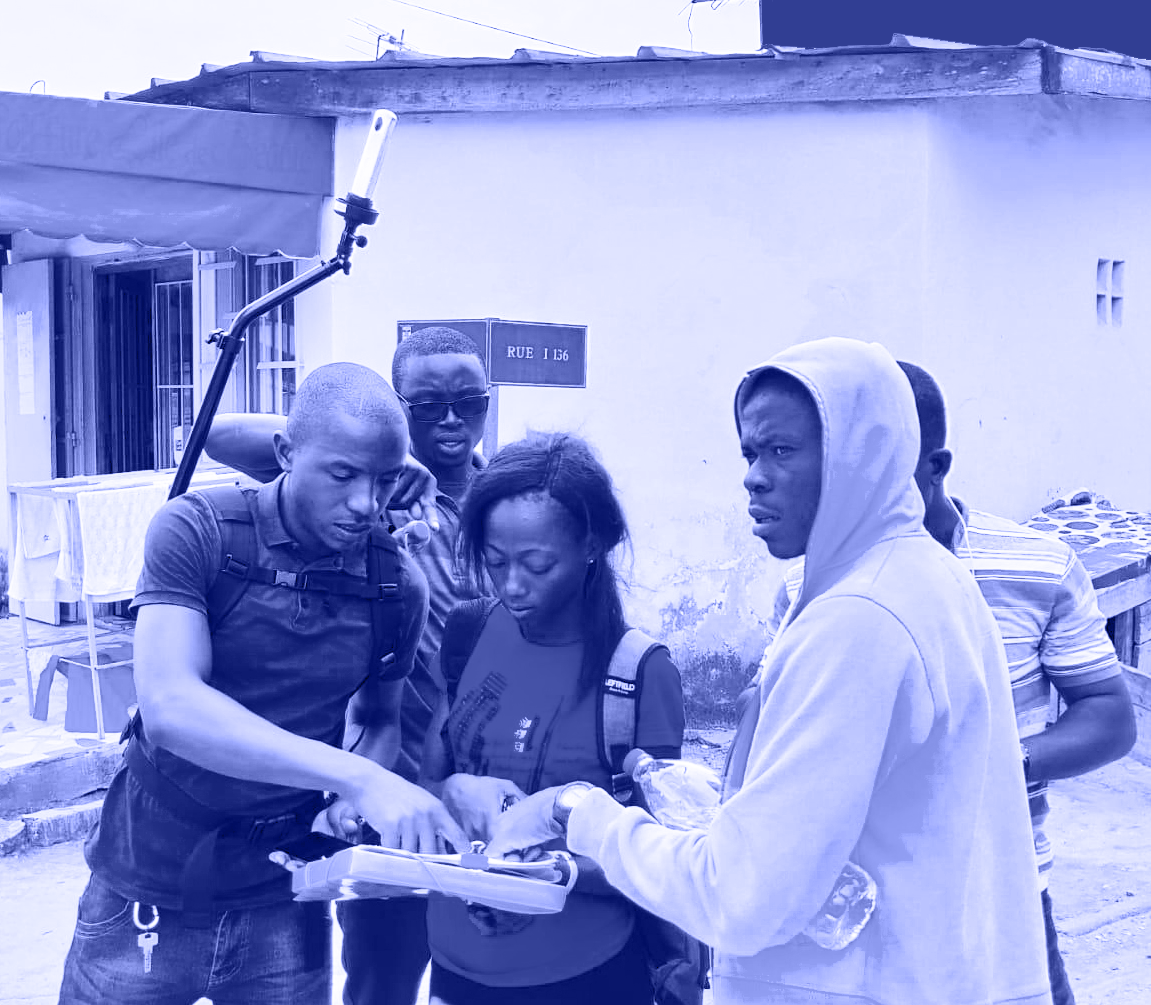
- Community mapping with street-level photo survey and computer vision
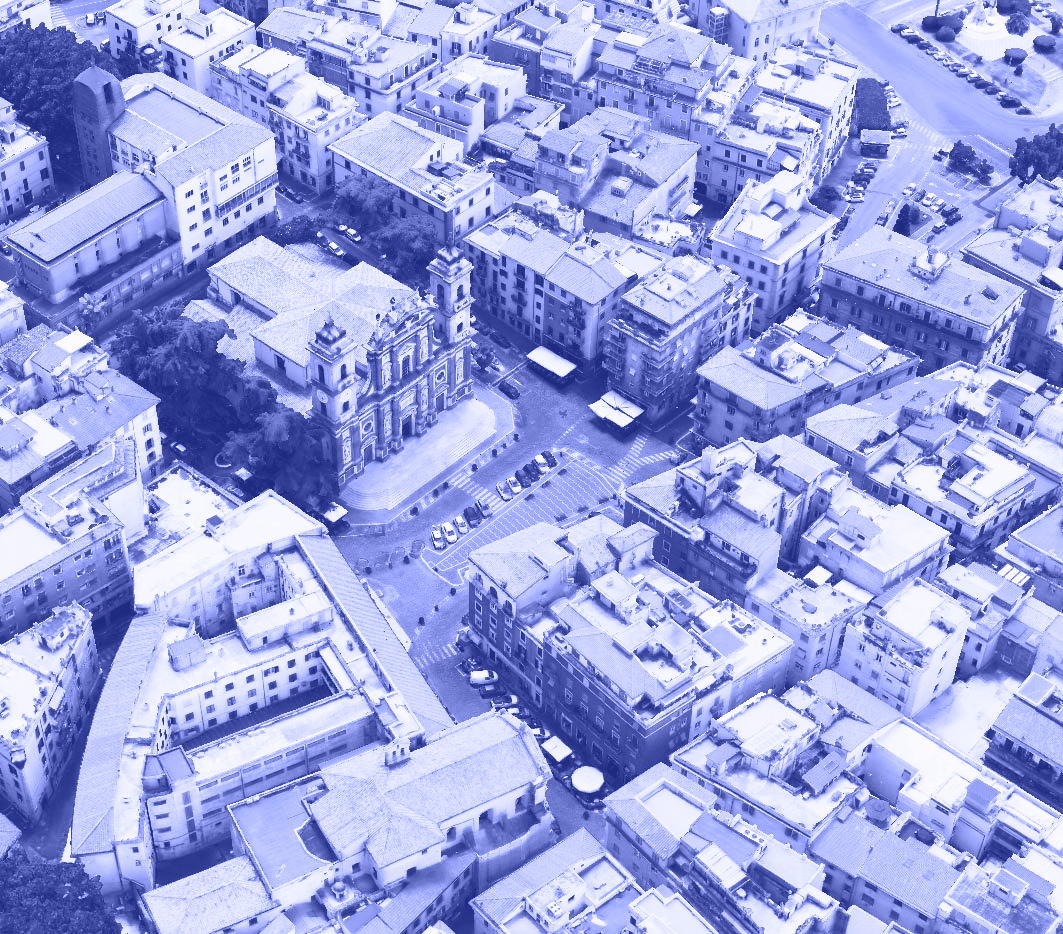
- Urban Digital Twins: shaping the future of smart sustainable cities
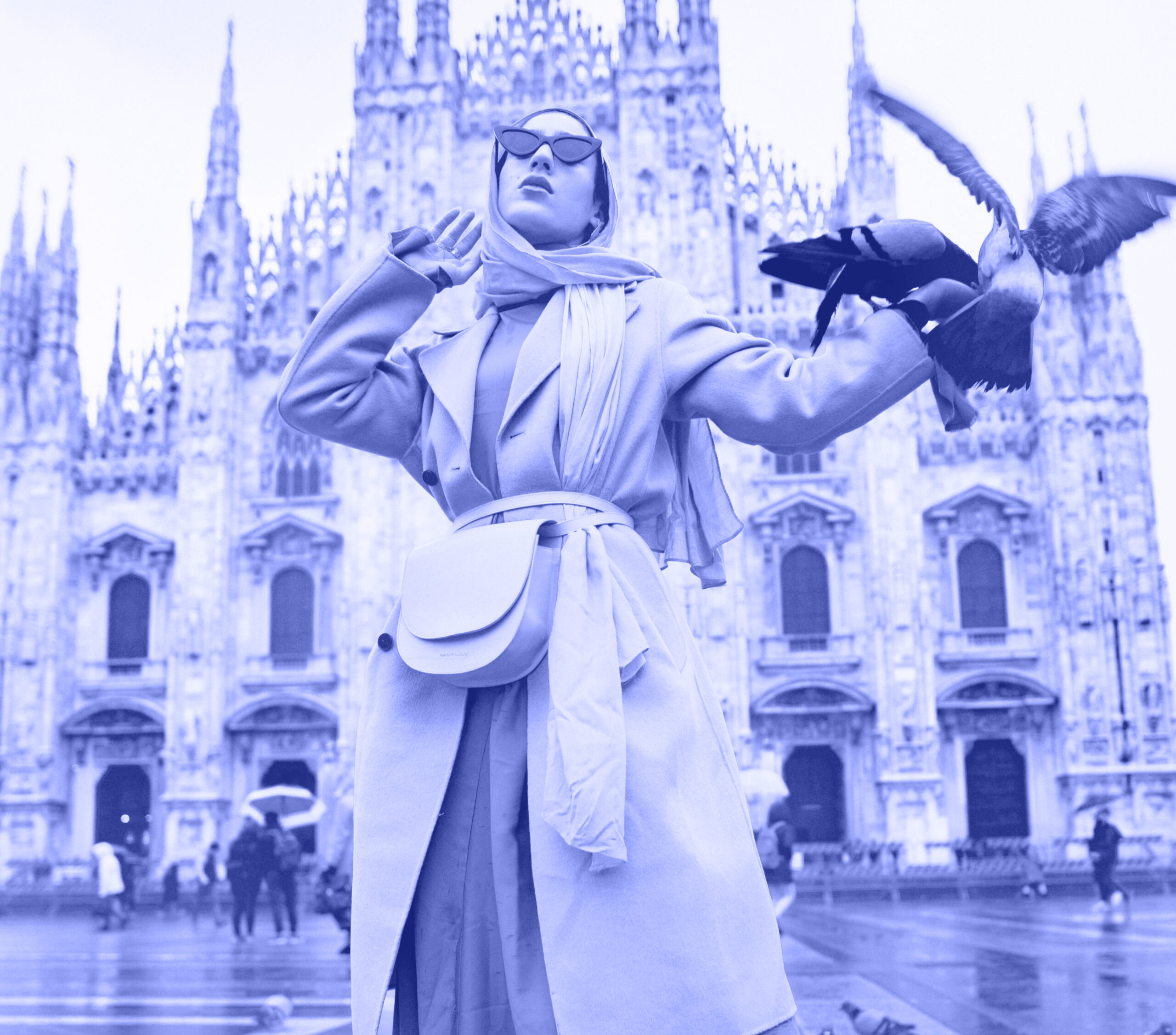
- Data-driven fashion trends from mobile mapping + Artificial Intelligence