Humanitarian and development
Community-based cartography & EO
for Urban Resilience
Combining community-based mapping and Earth Observation data
to support Urban Resilience Transitions in Côte d'Ivoire
Overview
Côte d'Ivoire's rapid economic growth and urbanization have led to increased poverty in urban areas and severe environmental issues, particularly in Abidjan. This expanding and thriving city faces significant challenges due to inadequate drainage systems, poor waste management, and hazardous land use. These factors heighten Abidjan's vulnerability to natural disasters, such as floods, which are becoming more and more frequent.
Funded by the World Bank, the "Community Mapping and Nature-Based Solutions project in Abidjan" uses innovative methods to mitigate flood risks and improve waste management. At MindEarth, we have partnered with OpenStreetMap Côte d'Ivoire (OSMCI), UrbaPlan, and Sepia Conseils to support Abidjan's development towards urban resilience through advanced geospatial processing techniques and community-based cartography.
Building reliable data from the ground up
At MindEarth, we harness the power of IoT to create new data with the help of local community and tackle urban and environmental challenges head-on. For this activity, Our innovative approach integrates various bottom-up data collection approaches, including street-level photo imagery, air quality sensors, and micro-tasking to provide a new, granular persepctive on urban environments.
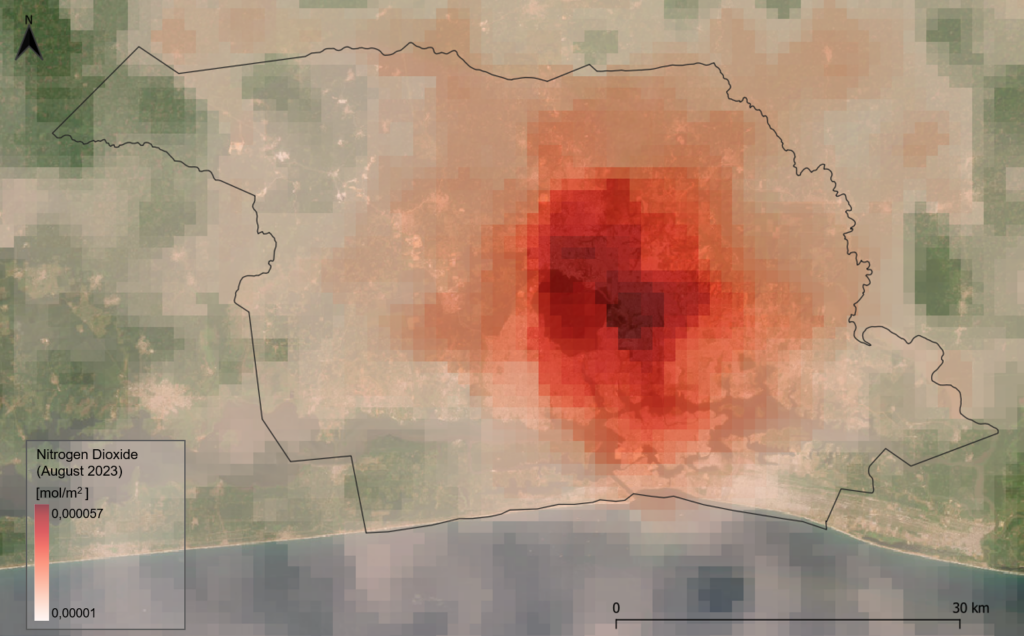
Street-level photo streams
from any smartphones
With the MindEarth app, users transform their smartphones into powerful data collection tools. As they walk through urban areas, they capture and upload street-level imagery, documenting critical elements like vegetation and litter. Each image is precisely geotagged using the device’s camera and GPS, ensuring accurate location data. The app’s automatic privacy protections blur faces and license plates, complying with privacy regulations. This community-driven method not only enriches our data with extensive geographic coverage but also empowers citizens to play an active role in urban and environmental initiatives.
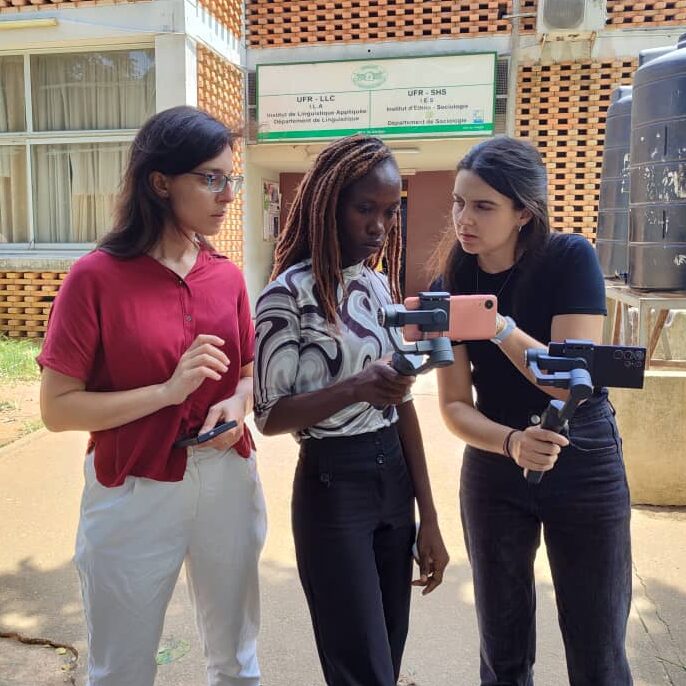
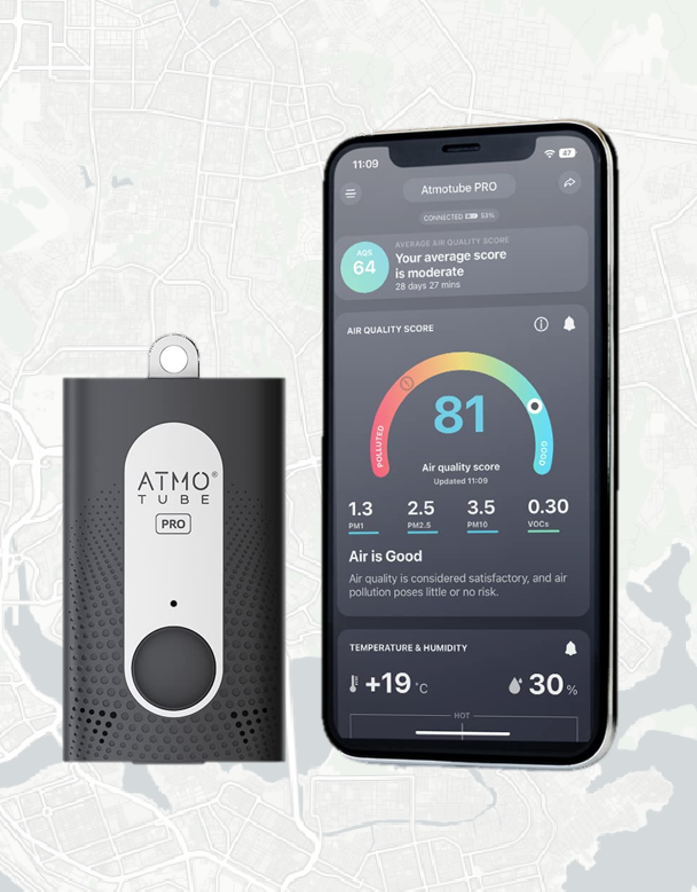
Air quality monitoring with wearable sensors
Our use of AtmoTube Pro portable environmental sensors sets a new standard in field mapping. These sensors collect vital data on temperature, air quality, particulate matter (PM1, PM2.5, and PM10), volatile organic compounds (VOC), humidity, and pressure. The data is automatically synchronized with the cloud, allowing us to analyze pollutant distribution over time. This real-time, high-resolution data collection enables us to identify pollution hotspots and track environmental changes with precision.
Microtasking for street-level imagery annotation
The imagery collected through the MindEarth app undergoes a detailed microtasking annotation process. We generate segmentation masks from a subset of the images, categorizing types of waste such as construction material, plastic, and metal. These annotations are crucial for creating models for the automatic extraction of waste from imagery, tailored specifically to Central African cities where training data on this types of features is scarce. This enhances our ability to understand waste distribution and supports targeted environmental interventions.
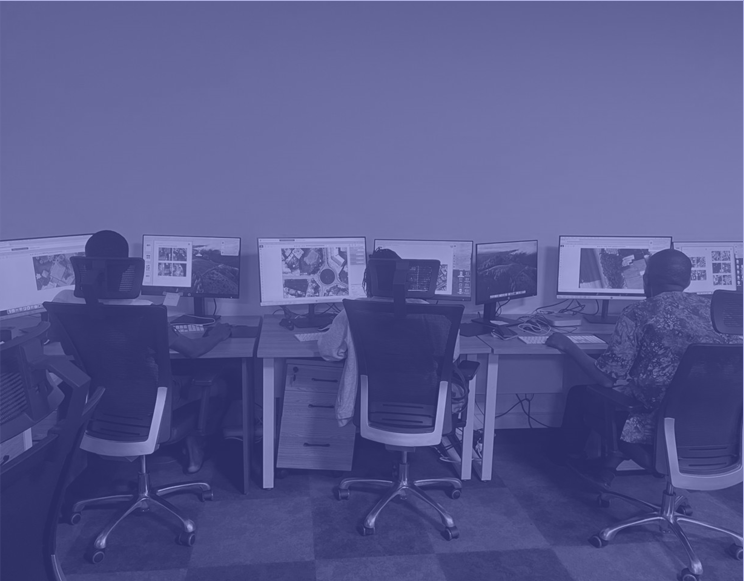
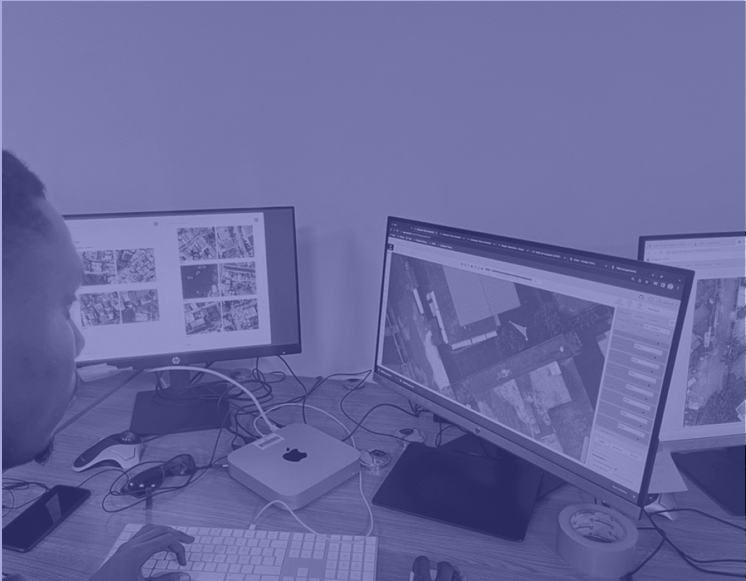
Urban planning insight from the space down
Earth Observation data is key in this project, enabling us to understand large-scale dynamics across Abidjan’s urban environment environment. It provides consistent, high-resolution data that reveals trends and changes over longer periods of time, crucial for informed and strategic decision-making.
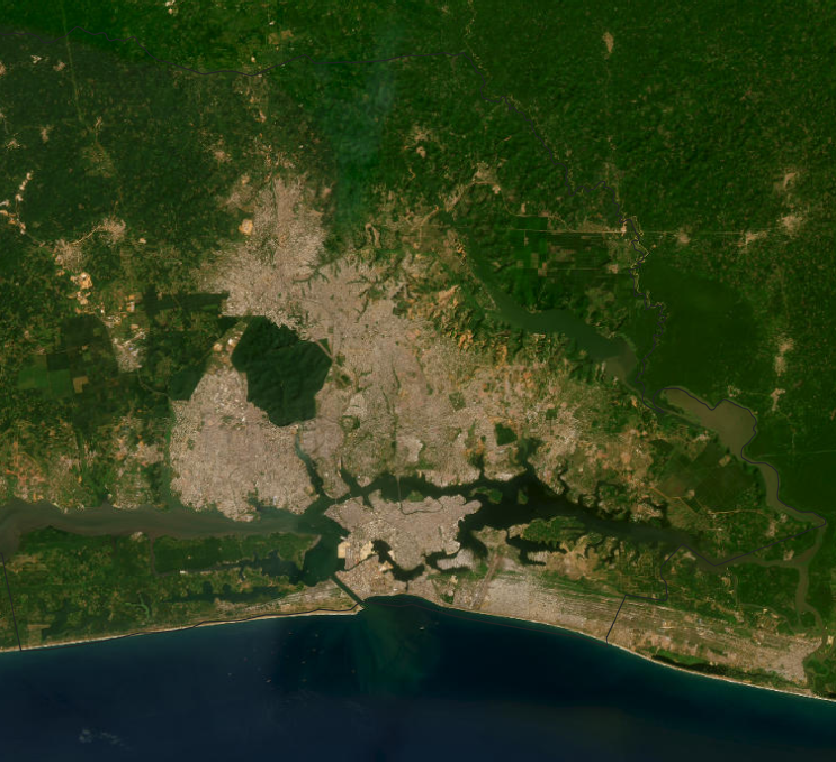
Vegetation health
We used an advanced algorithm that accounts for daily variations in cloud cover to produce yearly Normalized Difference Vegetation Index (NDVI) data at a 10m resolution for Abidjan city. This high-resolution data layer allows urban planners to monitor and assess the health and density of vegetation over time across the city, providing valuable insights into urban greenery and its changes throughout the year.
Day and night urban heat
Our analysis of Land Surface Temperature (LST) involved compiling monthly average day and night temperature layers for the entire Côte d’Ivoire from 2016 to 2023. This comprehensive data set captures the temporal variability of temperatures, helping us understand seasonal patterns and urban heat island effects. These temperature layers are crucial for studies related to climate change, urban planning, and environmental monitoring.
Air pollutant concentration
We analyzed Sentinel-5P TROPOMI data to calculate monthly concentrations of six key pollutants—Nitrogen Dioxide (NO2), Ozone (O3), Formaldehyde (HCHO), Sulphur Dioxide (SO2), Carbon Monoxide (CO), and Methane (CH4) for Côte d’Ivoire between 2018 and 2023. These data layers reveal the spatial and temporal distribution of air pollutants, providing essential information for air quality management and environmental policy-making.
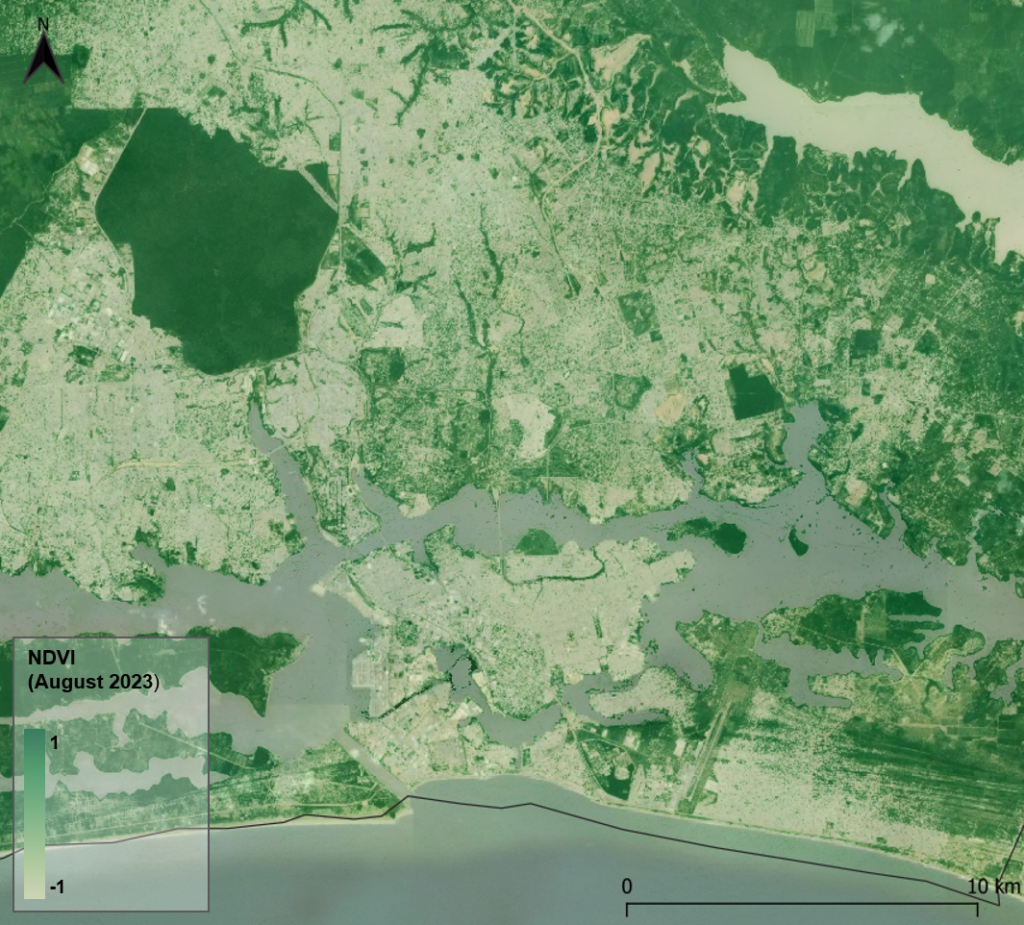
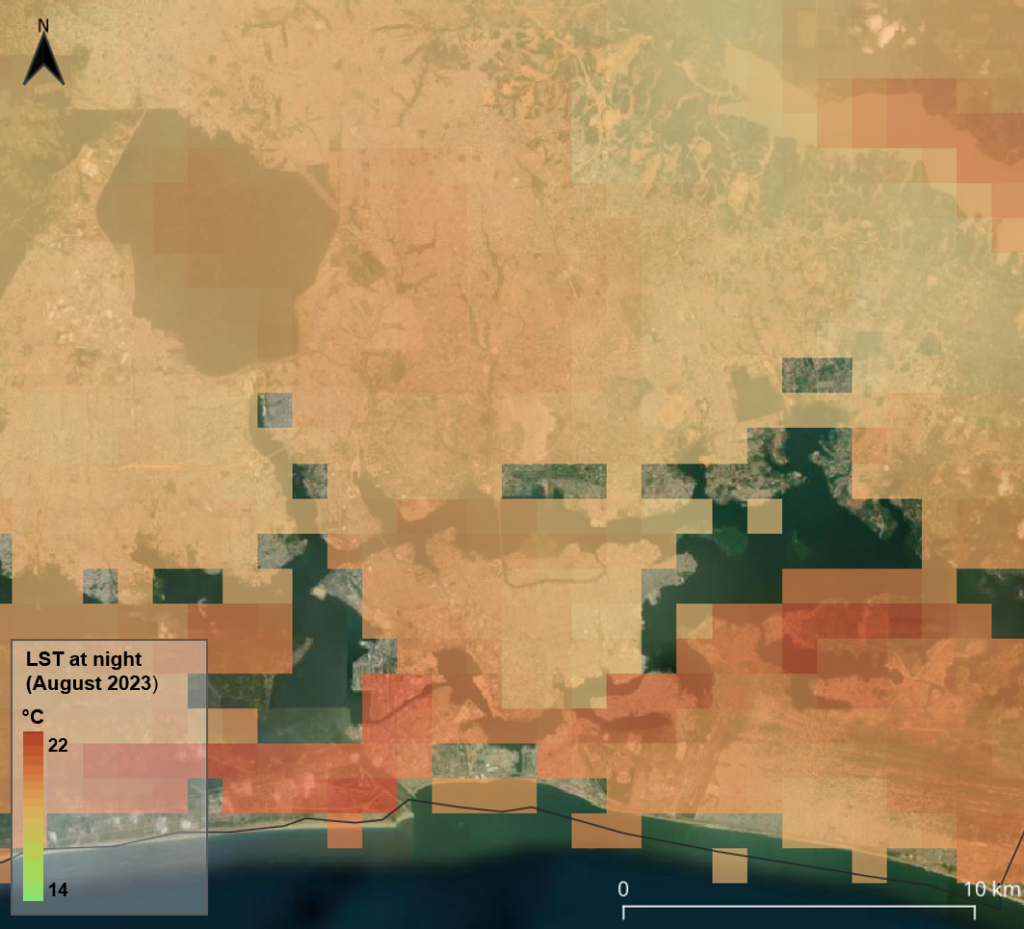
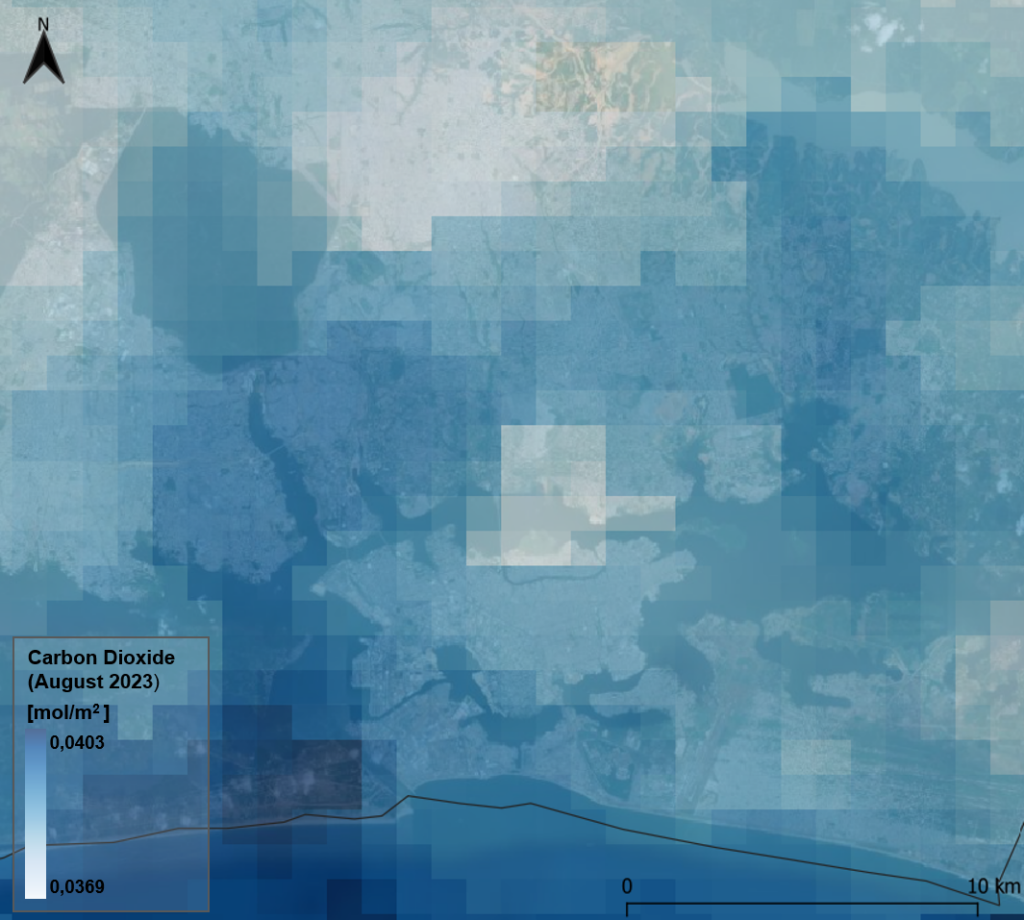
Crowdmapping in African Cities: Moving Forward
Workshops and training sessions were carried out for developing skills in digital mapping, sensor technology, and the integration of AI tools in community mapping. These activities were conducted at the Université Félix Houphouët-Boigny and PARU (Projet d’Assainissement et de Résilience Urbaine), where participants gained practical field experience by using sensors and a mobile application to collect and analyze data.
Single tree detection
To identify individual trees in urban areas we used a high-resolution (5 cm) orthophoto of the city of Abidjan. We applied a semi-automatic segmentation method and generated annotated datasets for the detection of single trees, including their crown area. This data layer reveals the spatial distribution of trees in the city, crucial information for monitoring vegetation presence and patterns and in urban areas.
Identifying waste
We used street view imagery to identify different types of waste commonly found in Abidjan such as plastic, construction material, metal, and mixed waste. A library of annotated images was generated, showcasing the spatial distribution of waste types. This dataset provides valuable input for training machine learning models aimed at automating waste detection for supporting monitoring and effective waste management.
Air pollution concentration
We measured air pollution in urban areas using Atmotube Pro devices deployed along city circuits to monitor particulate matter levels. Leveraging AI models, we scaled this data to cover the entire city, creating a comprehensive map of air pollution distribution on a typical day in Abidjan. This approach provides valuable insights for supporting decision-making for urban planning and public health initiatives.
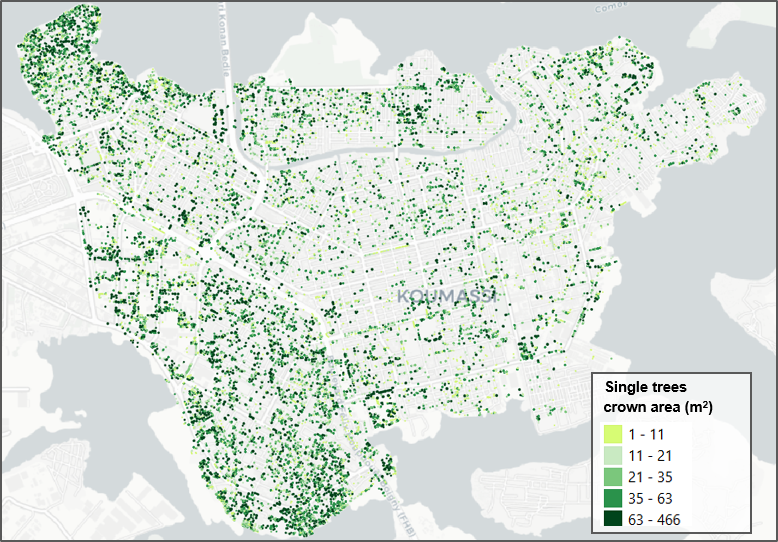
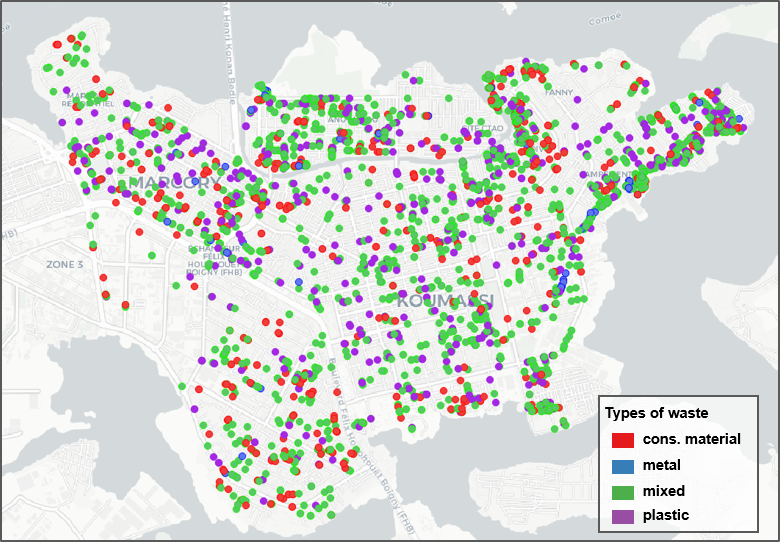
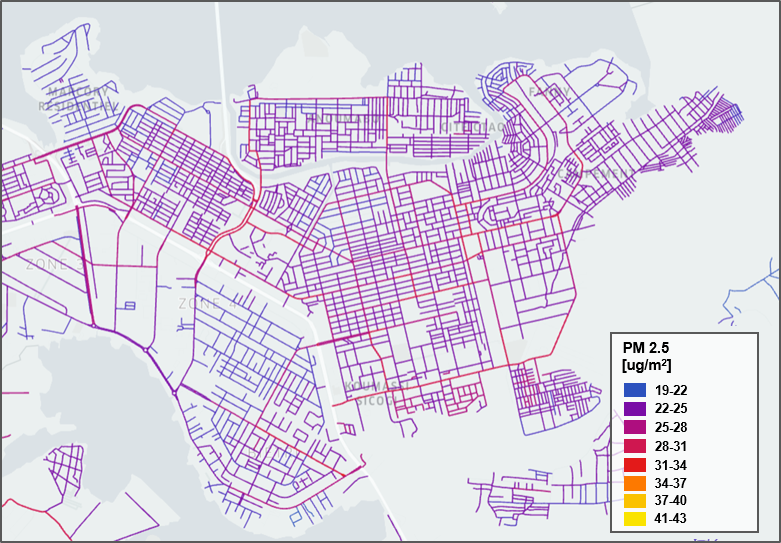
Explore more use cases
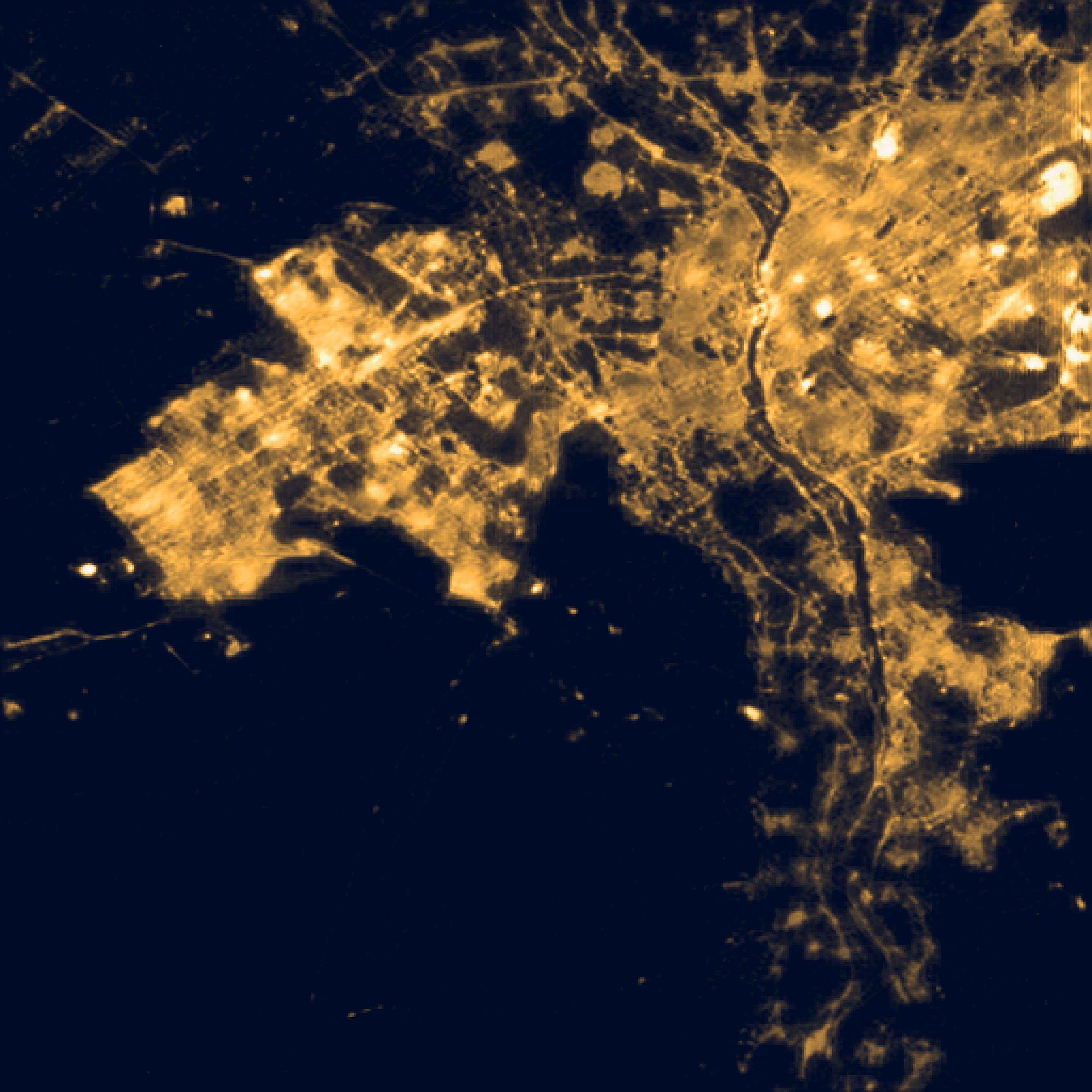
- SupR-NTL: Super-Resolution Nighttime Light for Sustainable Development
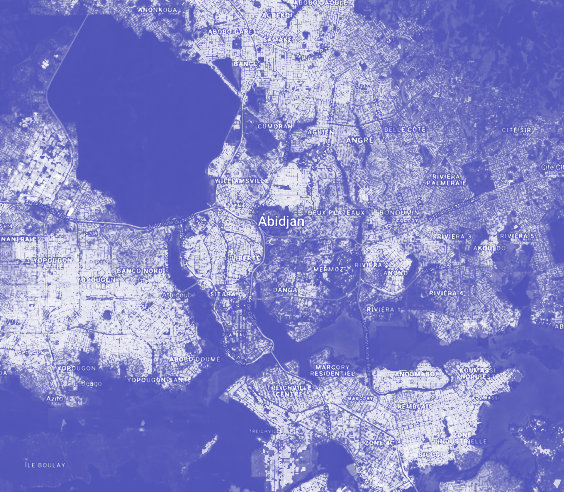
- Community-based cartography & EO for Urban Resilience
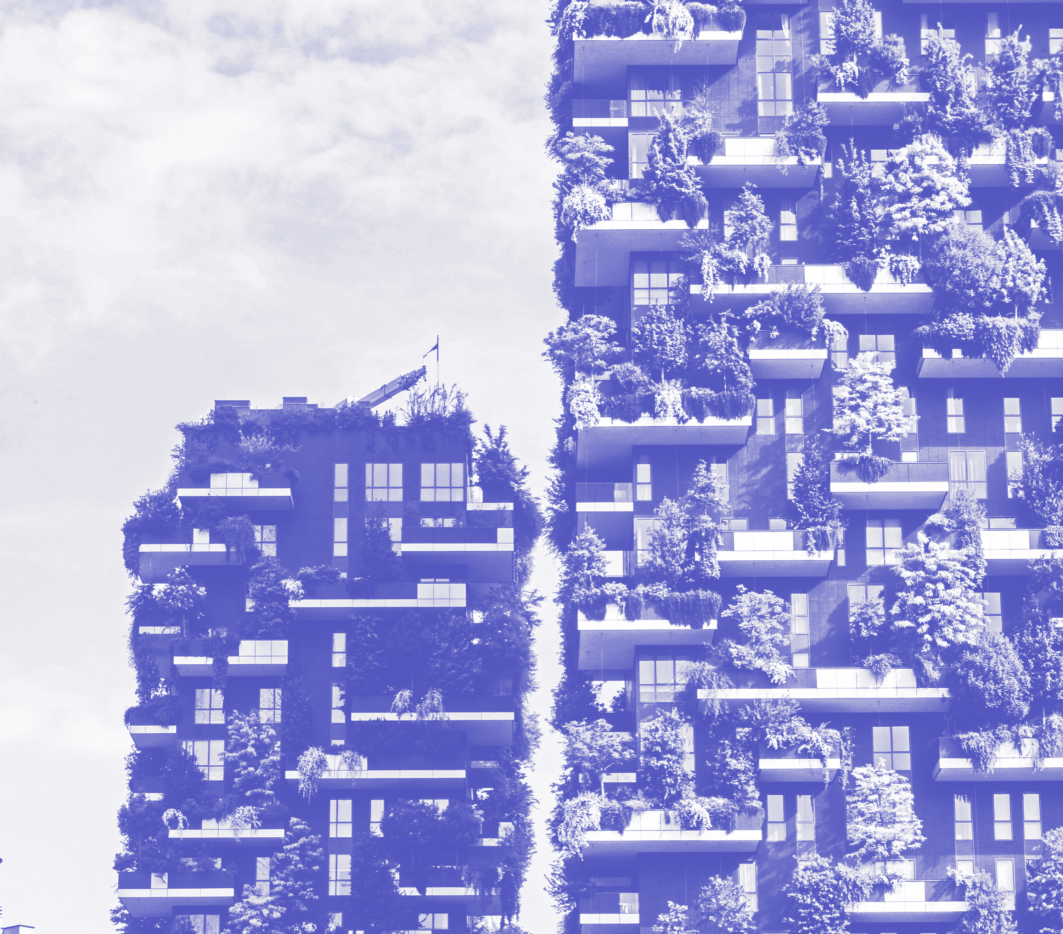
- BEE-AI: Building Energy Efficiency with Artificial Intelligence
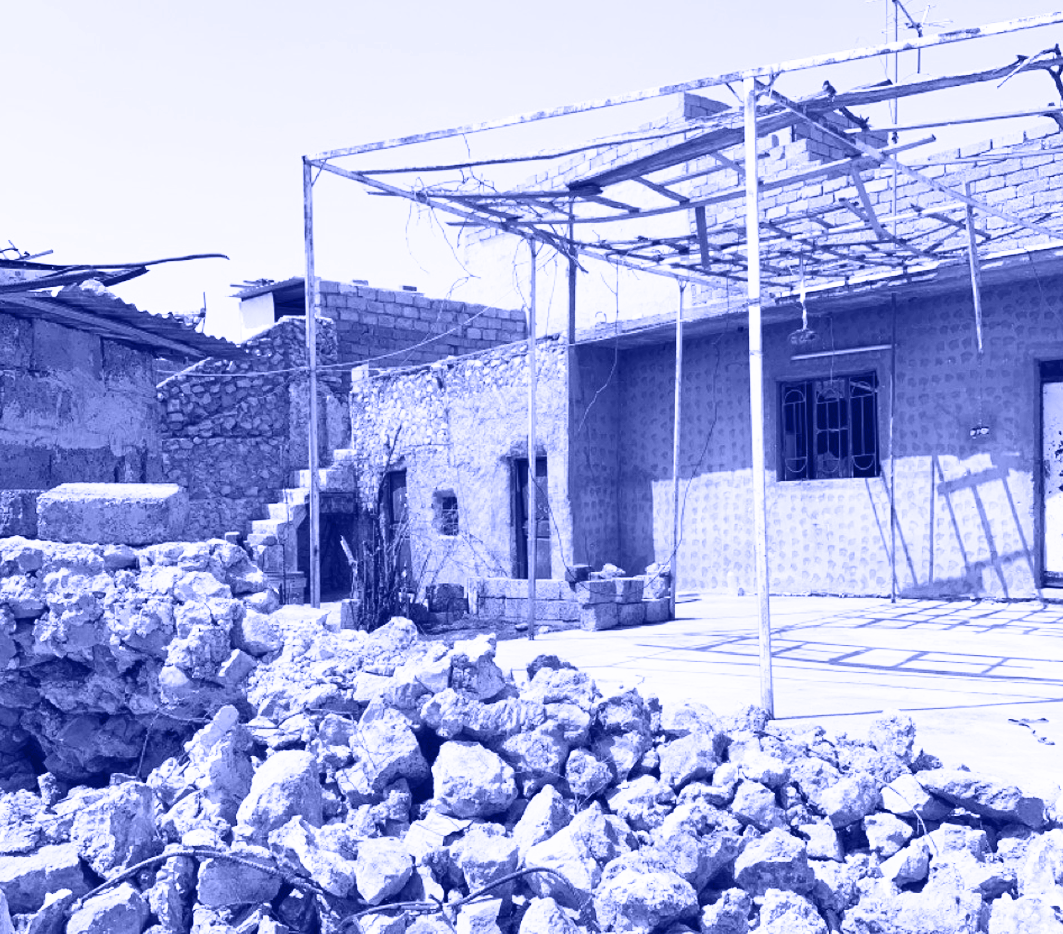
- Mapping the impact of armed conflict through Earth Observation
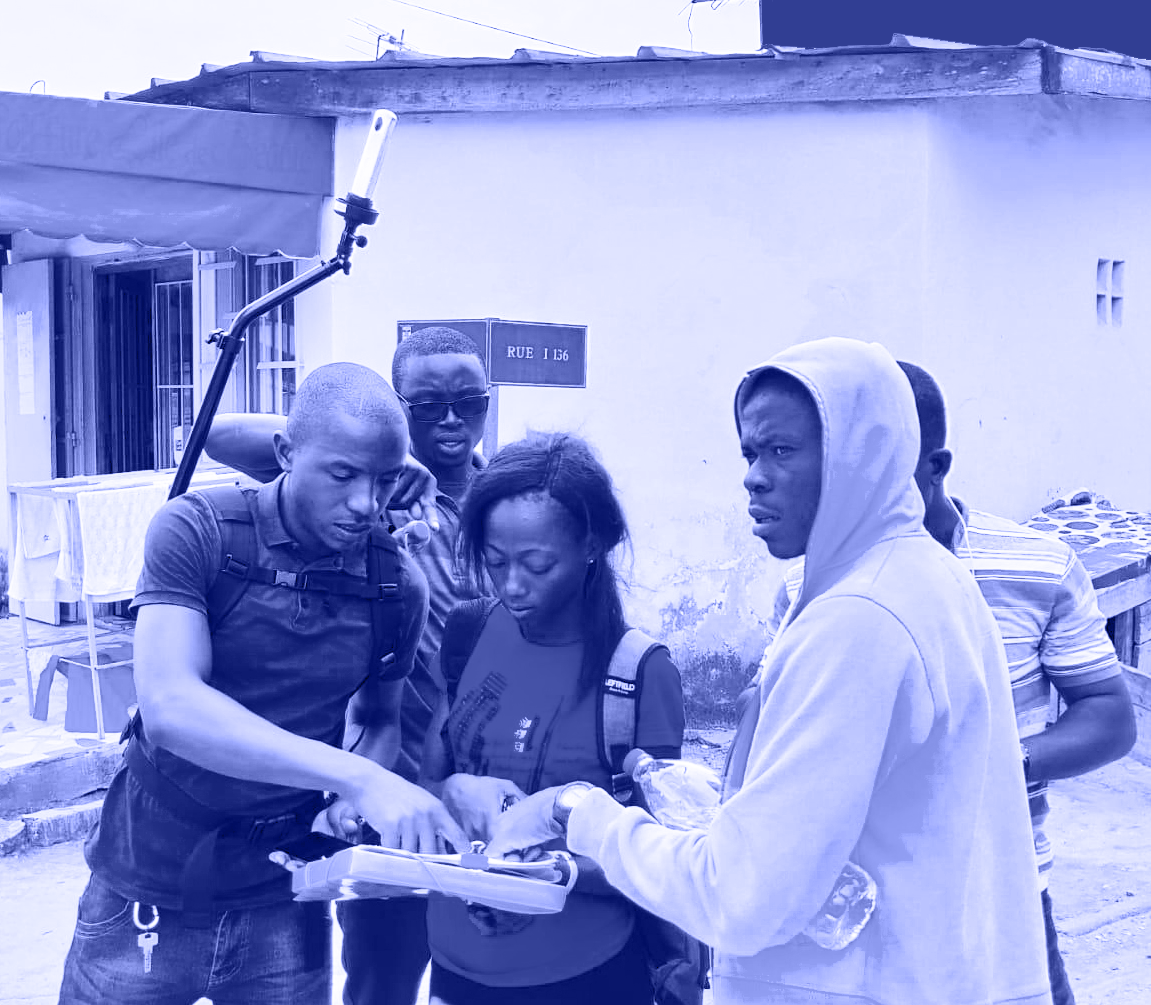
- Community mapping with street-level photo survey and computer vision
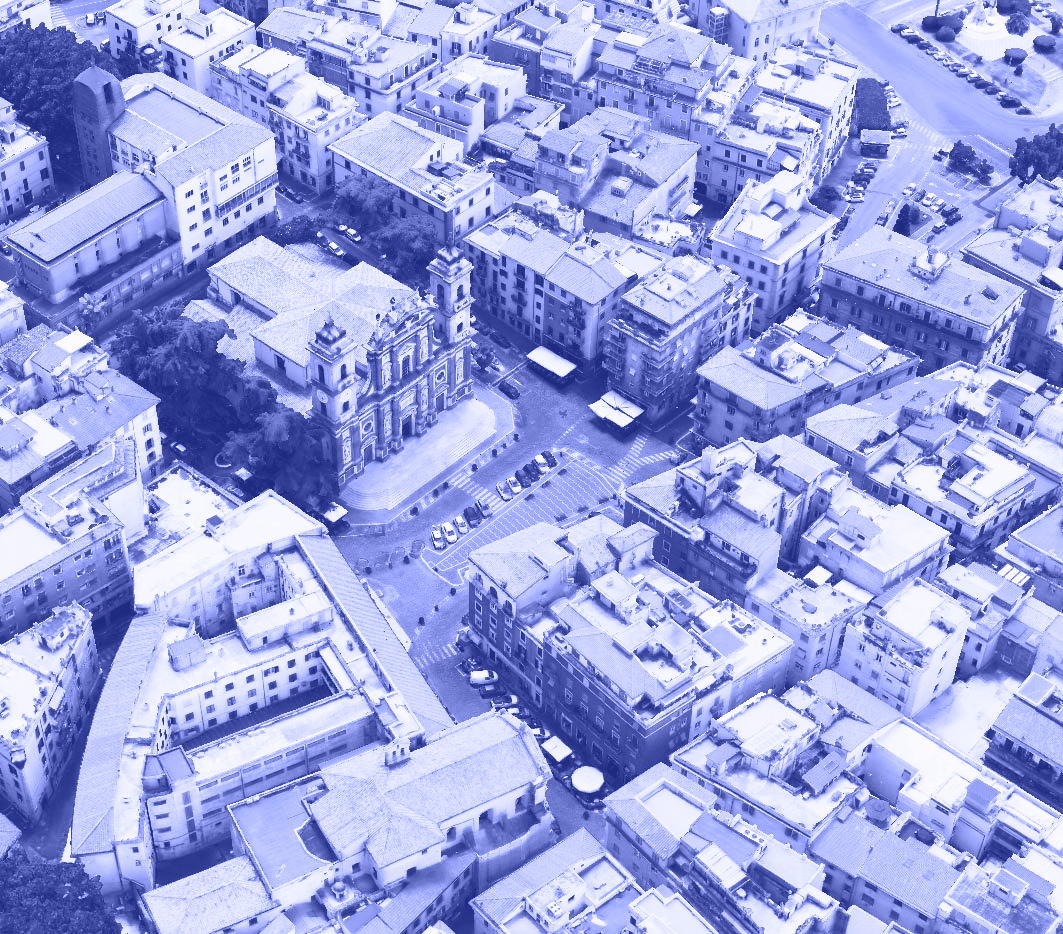
- Urban Digital Twins: shaping the future of smart sustainable cities
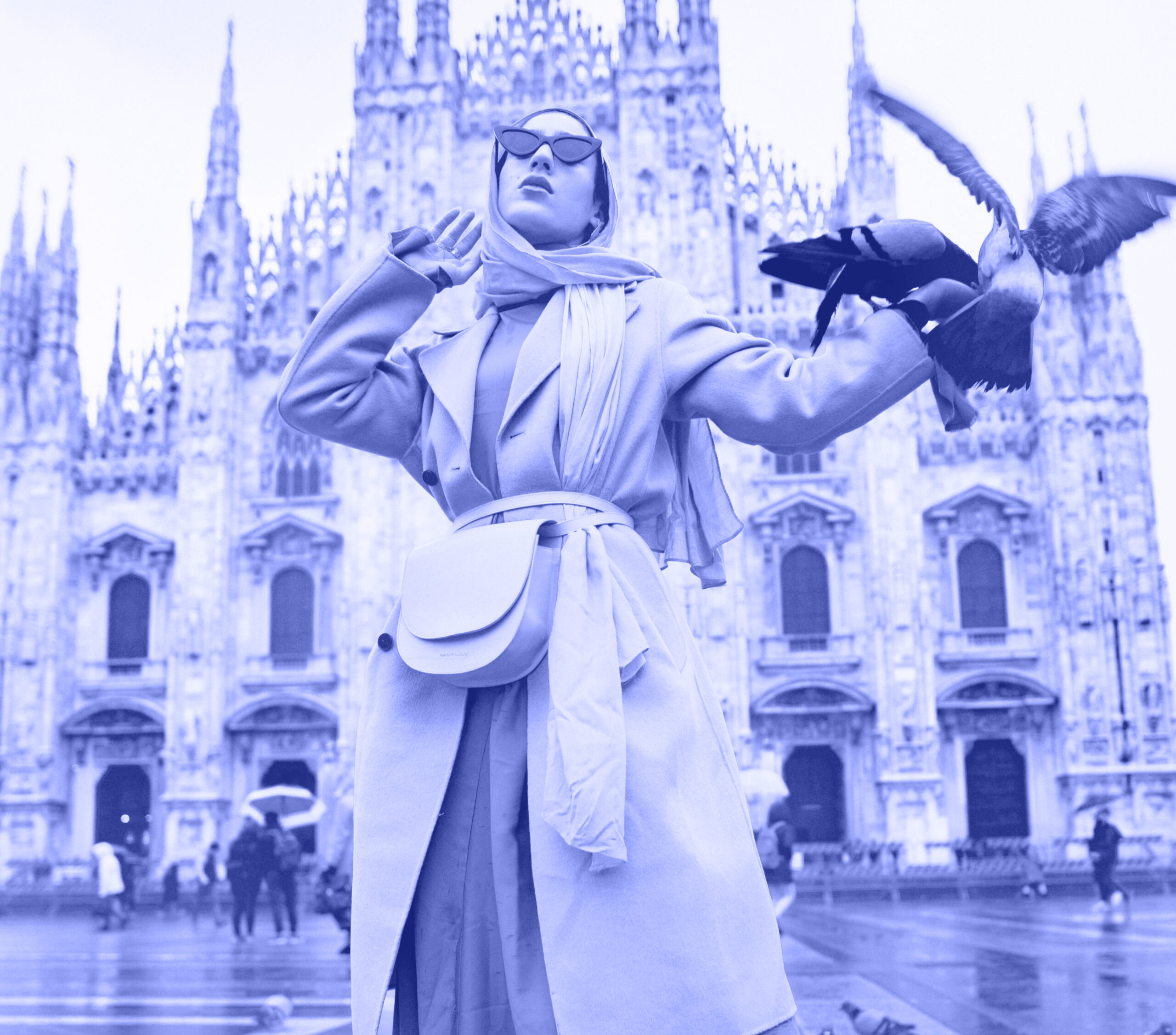
- Data-driven fashion trends from mobile mapping + Artificial Intelligence